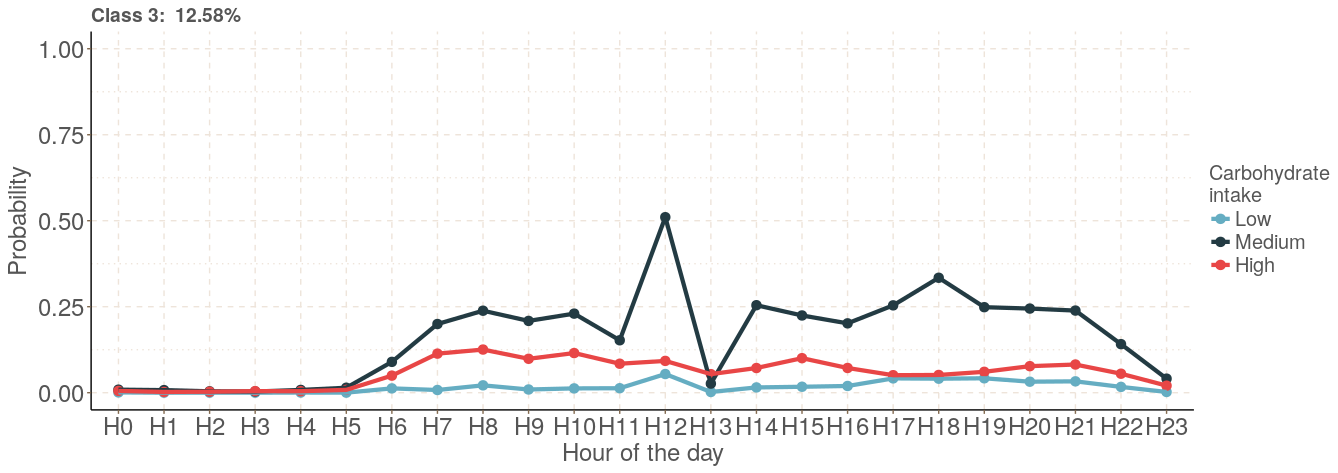
Words, notes, and sentences that may be useful
Words
- discernable [di’sə:nəbl, -’zə:-]
===== 辞典翻译: discernable ======
adj. 可辨别的;可认识的
============ 网络释义 ============
-------- discernable ---------
可辨别的
方向
分辨
-- discernable recognizable --
可辨别的
--- discernable visible ----
可辨别的
- abstinence [’æbstinəns]
====== 辞典翻译: abstinence ======
n. 节制;节欲;戒酒;禁食
============ 网络释义 ============
--------- abstinence ---------
节制
禁欲
禁戒
----- alcohol abstinence -----
酒戒断
----- Abstinence theory ------
节欲论
弃权
忍欲说
- exhaustive [iɡ’zɔ:stiv]
====== 辞典翻译: exhaustive ======
adj. 详尽的;彻底的;消耗的
============ 网络释义 ============
--------- Exhaustive ---------
无遗
详尽的
全部
----- Exhaustive search ------
穷举搜索
穷举搜索完全搜索
全程搜索
----- exhaustive voting ------
淘汰投票
消耗性投票
- temperament [’tempərəmənt]
===== 辞典翻译: temperament ======
n. 气质,性情,性格;急躁
============ 网络释义 ============
-------- temperament ---------
气质
气质 (心理学)
性格
------ Well Temperament ------
Well temperament
Well Temperament
平均律
---- Musical temperament -----
律学
一份音乐的气质
音乐性
- trivial [’triviəl]
======= 辞典翻译: trivial ========
adj. 不重要的,琐碎的;琐细的
============ 网络释义 ============
---------- trivial -----------
琐碎的
微不足道的
小巫见大巫
------ Trivial Pursuit -------
棋盘问答
打破砂锅问到底
追根究底
-------- trivial name --------
惯用名
俗名
种名
- prudent [’pru:dənt]
======= 辞典翻译: prudent ========
adj. 谨慎的;精明的;节俭的
n. (Prudent)人名;(法)普吕当
============ 网络释义 ============
---------- prudent -----------
谨慎的
明智的
审慎的
--------- prudent s ----------
善于经营的
谨慎
精明的
----- PRESIDENT PRUDENT ------
普鲁登特总统城
- daunting [’dɔ:ntiŋ]
======= 辞典翻译: daunting =======
adj. 使人畏缩的;使人气馁的;令人怯步的
============ 网络释义 ============
---------- Daunting ----------
令人生畏
使人畏缩的
使人气馁的
------ However Daunting ------
但艰巨
--------- B daunting ---------
使胆怯
- discern [di’sə:n, -’zə:n]
======= 辞典翻译: discern ========
vt. 识别;领悟,认识
vi. 看清楚,辨别
============ 网络释义 ============
---------- discern -----------
看出
辨别
识别
-------- Discern Lies --------
辨知谎言
辨识谎言
洞悉谎言
------- discern safely -------
安全识别
Expressions
Sentences
terry2017discontinuous
(Discontinuous Patterns of Cigarette Smoking From Ages 18 to 50 in the United States: A Repeated-Measures Latent Class Analysis)
RMLCA models were fitted in SAS 9.4 using PROC LCA. Parameters were estimated by maximum likelihood using the EM algorithm.
To ascertain if the same latent class structure was observed for males and females, multiple-group RMLCA models by sex were fitted in PROC LCA using the GROUPS statement (both with and without imposing measurement invariance across males and females).
Model selection (ie, the number of latent classes specified) was determined by model fit, parsimony, and stability.
Simulations have shown that the Bayesian information criterion (BIC) and sample size-adjusted BIC (a-BIC) perform particularly well at selecting the “correct” latent class model.
Improvement in both BIC and consistent Akaike information criterion (CAIC) values continued only through the 12-class model; thus, the 12-class model was selected as optimal.
lanza2007proc
(PROC LCA: A SAS procedure for latent class analysis)
In traditional LCA, two sets of parameters are estimated: class membership probabilities and item-response probabilities conditional on class membership.
Latent class models usually involve categorical indicators (although a version of LCA involving continuous indicators called latent profile analysis [Gibson, 1959] is being used increasingly).
When categorical data are used, the latent class model has the advantage of making no assumptions about the distributions of the indicators other than that of local independence; that is, the assumption that within a latent class the indicators are independent.
In PROC LCA, parameters are estimated by maximum likelihood using an EM (expectation-maximization) type procedure. Missing data on the latent class indicators are handled in this procedure, with data assumed to be missing at random (MAR).
A test of the null hypothesis that data are missing completely at random appears in the output.
a helpful preliminary step in any LCA is exploring overall relations among pairs of items by conducting cross-tab analyses.
A good starting point for identifying an optimal baseline model is to fit a sequence of models with two classes, three classes, and so on.
A variety of tools can be used together for model selection, including the likelihood-ratio G 2 statis- tic, Akaike’s Information Criterion (AIC; Akaike, 1974) and Bayesian Information Criterion (BIC; Schwarz, 1978).
For example, each class should be distinguishable from the others on the basis of the item-response probabilities, no class should be trivial in size (i.e., with a near-zero probability of membership), and it should be possible to assign a meaningful label to each class.
The AIC and BIC are penalized log-likelihood model information criteria that can be used to compare competing models (e.g., models with different numbers of latent classes) fit to the same data. A smaller AIC and BIC for a particular model suggests that the trade-off between fit and parsimony is preferable.
Often when a grouping variable is included it is important to test for measurement invariance across groups. To do this, a model with free estimation of the \(\rho\) parameters can be compared to the same model that includes restrictions equating the \(\rho\) parameters across groups. A significant p value suggests that the null hypothesis of measurement invariance should be rejected.
Based on a data set, a particular model specification, and starting values for the parameters, the algo- rithm iterates between the Expectation (E) step and the Maximization (M) step until either the convergence criterion is achieved or the maximum number of iterations is reached.
The best way to detect identification problems or local optima (i.e., solutions other than the optimal one) is to fit the same model using multiple sets of starting values. This can be done by calling the procedure repeatedly with different seeds specified.
Even well-identified models can land on a different solution occasionally; if the solution with the smallest log-likelihood is arrived at using the majority of the seeds, one can have confidence that it is the optimal solution.
collins2010latent
(Latent Class and Latent Transition Analysis: With Applications in the Social, Behavioral, and Health Sciences)
It is particularly noteworthy that the causal flow is from the latent variable to the indicator variable, not the other way around. That is, observed indicator variables do not cause the latent variables.
The purpose of LCA is to help the investigator to discern any meaningful, scientifically interesting classes against the noisy background of error.
In LCA, it is the responsibility of the investigator to assign names to the latent classes.
The starting point for conducting a latent class analysis on empirical data is a contingency table formed by cross-tabulating all the observed variables to be involved in the analysis.
The local independence assumption refers only to conditioning on the latent variable. It does not imply that in a data set that is to be analyzed, the observed variables are independent. In fact, it is the relations among the observed variables that are explained by the latent classes.
An observed data set is a mixture of all the latent classes. Independence is assumed to hold only within each latent class, which is why it is called “local”.
LCA makes the assumption of local independence, which states that conditional on latent class, observed variables are independent.
A high degree of latent class separation implies a high degree of homogeneity.
However, a high degree of homegeneity does not necessarily imply a high degree of latent class separation.
Thus, it is possible to have high homogeneity but poor latent class separation.
Some of the latent classes may be characterized by more than one response pattern, but there is no response pattern that appears to be closely associated with more than one latent class. Thus the latent classes are conceptually distinct and can readily be labeled.
Model selection may be challenging. Furtunately, a variety of tools are available to assist, including tests of absolute model fit, assessment of relative fit of competing models, and cross-validation. In addition to the tools discussed, there are two additional consideration that are critically important when evaluating a model: parsimony and model interpretability.
We see statistical models as lenses through which investigators examine their data in order to gain useful insights.
The likelihood-ratio statistics \(G^2\) \[G^2 = 2\sum_{w=1}^W f_w\log(\frac{f_w}{\widehat{f_w}})\]
Where \(f_w\) represents the observed frequency of cell \(w\), and \(\widehat{f_w}\) represent the expected frequency of cell \(w\) according to the model that has been fit.
The larger the value of \(G^2\), the more evidence there is against the null hypothesis. (The larger it is, the worse model fitting it is.)
The degrees of freedom of \(G^2\): \[df = W - P - 1\]
Where \(P\) is the number of estimated parameters.
Sometimes fitting a series of latent class models with different numbers of latent classes to data at a single time point can be helpful as a preliminary step in model selection in LTA. Doing this at each time point can be informative about the latent structrue within times, and how that structure changes accross timie points.
It is important to note that the best-fitting latent class model at any given time may not correspond to the best-fitting latent transition model fit to all occasions of measurements. The best-fitting model based on the data from all occasions of measurement may include a different number of latent stuatuses than the number of latent classes identified at one particular time.
However, based on the latent status prevalences at each time alone, it is impossible to tell hou and to what extent individuals were moving between latent statuses.
In 7.11 we used a different empirical example to test the hypothesis that the item-response probabilities in a LTA are equal across times. This type of restriction on the item-response probabilities is commonly used in LTA. It helps with model identification and, importantly, ensures that the meaning of the latent statuses remains constant over time.